- Yvonne Huiqi Lu
- Feb 6, 2024
- 2 min read
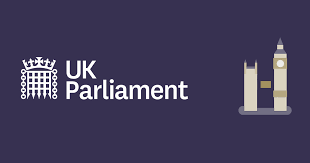
On 2 February, 2024, the UK Parliament published the report on large language models (LLMs) and generative AI (GAI). Main take-away messages were summarised by the Committee as follows:
The Committee considered the risks around LLMs and says the apocalyptic concerns about threats to human existence are exaggerated and must not distract policy makers from responding to more immediate issues.
The report commented on near-term security risks of LLMs including cyber attacks, child sexual exploitation material, terrorist content and disinformation. The Committee says catastrophic risks are less likely but cannot be ruled out, noting the possibility of a rapid and uncontrollable proliferation of dangerous capabilities and the lack of early warning indicators. The report called for mandatory safety tests for high-risk models and more focus on safety by design.
The Committee calls on the Government to support copyright holders. It rebukes tech firms for using data without permission or compensation.The report calls for a suite of measures including a way for rightsholders to check training data for copyright breaches, investment in new datasets to encourage tech firms to pay for licensed content, and a requirement for tech firms to declare what their web crawlers are being used for.
The Committee sets out 10 core recommendations to boost opportunities, address risks, support effective regulatory oversight, introduce new standards, and resolve copyright disputes.
For complete report, please following this link: https://publications.parliament.uk/pa/ld5804/ldselect/ldcomm/54/5402.htm
Further readings: The EU’s AI Act at a Crossroads for Rights, Institute for Ethics in AI, University of Oxford.
In the next blog, I will share thoughts on the Ethics and governance of artificial intelligence for health: Guidance on large multi-modal models, published by the World Health Organisation on18 January 2024.